Embracing Edge AI: How to fuel growth with real-time decision-making
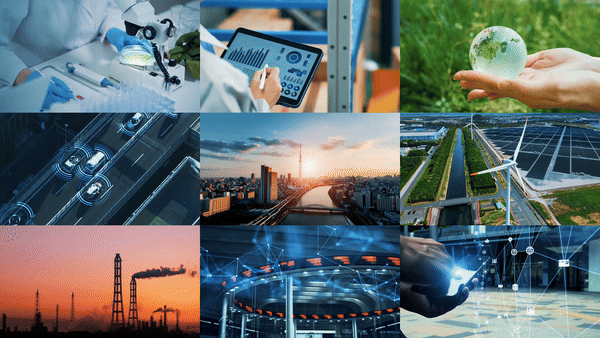
There is an artificial intelligence (AI) revolution happening, but it’s not in the cloud, and it doesn’t involve gigantic models that help you write term papers, create cool images, or generate sounds like a human voice. This revolution is a little closer to you—the user. It’s at the network edge in small devices like your smartwatch, smart appliances, cameras, automobiles, and factory machinery. It is edge AI.
What is edge AI?
At its most basic, edge AI is placing AI in applications near data sources or directly on local devices (like drones, cameras, smartwatches, and automobiles) that sense, monitor, and record their environment. Edge AI helps these local devices harness their processing power to perform tasks autonomously.
The bigger picture is that edge AI is about where processing occurs. With edge AI, the processing occurs right where data is collected. This means the sensor in your smartwatch, automobile, or factory machine can make precise, split-second decisions. If those same sensors have to send data back to the cloud or server for processing, latency occurs, which can undermine timely decisions. For some applications of edge AI, the split-second decision can mean life or death for the humans involved.
How is this different from AI in the Cloud?
Both cloud and edge AI share the goal of simulating human intelligence through data collection and processing. However, edge models run on devices at the network edge that may or may not be connected. Cloud AI models run in the always-connected cloud computing infrastructure. They tend to be large in size, requiring vast amounts of data to run effectively and use a massive amount of cloud computing resources. Conversely, edge AI is small, fast, and more efficient.
Edge AI, TinyML, or On-device Inference?
The industry has been using these terms synonymously, although they focus on different aspects of the AI that is trained and deployed. For example, using the term “edge” implies having processing outside the cloud, while the term “tiny” refers to the resource constraints on hardware that the AI is deployed on. The term “on-device” refers to the cohabitation between data and processing. In reality, all of these terms refer to the need to anticipate and adapt the AI training and deployment with utilized resources (size, weight, power, etc.) for inference.
Are edge devices the same as IoT devices?
The Internet of Things (IoT) devices and edge devices are different, but they can collaborate. IoT devices gather and send data using specialized software, connecting to the cloud or data center for data processing. Edge devices, on the other hand, perform computations locally and offer extra processing power and storage. This enables them to recognize patterns, provide immediate business insights, and make real-time decisions.
Why do I need edge AI?
The explosive adoption of cloud AI is causing organizations to strategically consider how they can exploit the power of AI to transform their business operations and disrupt competitors. They are quickly discovering that the cost of cloud AI is untenable in the long term, and the benefits of locating AI-powered applications at the functional edge will enable them to reduce costs, improve customer experiences, and disrupt their competitors. Scalability is a key differentiator because today’s cloud-centric solutions are facing limits in bandwidth, latency, and even power to sustain AI workloads. Forward-looking organizations are leaning into next-generation IT architectures that will take advantage of the edge continuum and move data processing closer to the source.
What are some of the use cases?
Applications for edge AI are characterized by their ability to leverage real-time data processing and analysis to drive efficiency, improve decision-making, and enhance user experiences across various industries.
Some of the most advanced use cases are found in national defense:
- Underwater threat detection: Computer vision models are used to help sailors clear commercial shipping zones or contested waters.
- Automatic Target Recognition: Machine learning models are employed to autonomously recognize and classify various targets, from vehicles to aircraft and personnel, in real-time, leaving the human operators to focus on critical tasks and rapidly make informed decisions.
- Airborne ISR: Several military entities maintain a tactical advantage against emerging threats with quicker data-to-decision and real-time results using edge AI on airborne intelligence, surveillance, and reconnaissance drones.
Edge AI can leverage geospatial data and support a variety of use cases:
- Emergency management: Disaster management experts look to AI for assistance in predicting, mitigating, and responding to natural disasters.
- Urban management and public safety: AI-driven applications can provide valuable insights into crowd density and movement, enhancing outcomes for community and government entities as they plan and monitor large sporting events, music concerts, and more.
- Agriculture and resource management: Edge AI can provide site-specific help in precision agriculture when sensors on drones and tractors are deployed to collect real-time data on soil conditions, crop health, and moisture levels.
Industry and manufacturing have several use cases for computer vision models using both cloud and edge AI:
- Predictive maintenance: Edge AI analyzes real-time sensor data to predict potential equipment failures, enabling proactive maintenance and extended equipment lifespan.
- Quality assurance: edge AI can detect anomalies, identify root causes, and implement corrective actions immediately, minimizing waste and rework.
- Worker safety: Edge AI can significantly enhance worker safety by tracking worker movements, monitoring the work environment for hazards, and triggering emergency actions.
Several business applications touch consumers on a daily basis:
- Smart homes: Controlling devices, optimizing energy consumption, and enhancing security.
- Wearable devices: Many consumers wear sensors on their wrist to help monitor health and exercise and provide personalized recommendations.
- Automobile driver assistance: Computer vision AI is enhancing driver safety with features like lane departure warning and collision avoidance.
What are the benefits of using Edge AI?
Edge AI offers unique advantages over other AI solutions by processing data closer to the physical world. This proximity allows edge AI to be more responsive, contextually aware, and independent. Edge AI can enhance the performance, efficiency, and security of AI applications with the following advantages,
Cost-effective
- Edge AI operates on smaller, more affordable devices that are easier and cheaper to maintain and replace compared to investing in extensive cloud infrastructure.
- Edge devices are low-power and often run on stored or renewable energy, which can be more cost-effective than the high energy usage of cloud-based solutions.
- Edge devices require little to no connectivity and lower bandwidth, which can translate to lower communication costs.
- Edge AI models are smaller and require less data to train because they can be customized to their environments. In contrast, cloud AI necessitates larger models and extensive datasets.
Real-time decision making
- Edge AI enables real-time solutions through low latency, whereas cloud systems are designed for high throughput which may compromise on latency.
- Edge AI generates, processes, and takes action locally without relying on system components that can slow down, such as low bandwidth or poor connectivity.
Resilience
- Edge AI models are built to be energy efficient, enabling them to run for extended periods using stored or local energy sources.
- Edge devices are resilient to network outages and can operate with intermittent or no network connectivity.
- Edge devices are inexpensive and compact, making them ideal for building redundancy to mitigate device outages.
Privacy and security
- Edge devices collect and process data locally. Therefore, they can be designed to prioritize privacy without sacrificing functionality.
- Edge devices can operate with intermittent connectivity and limited energy, making their security easier to manage compared to cloud-based systems with a larger attack surface.
- Edge devices are deployed on-premises, allowing users to implement more effective physical security measures. While cloud security is robust, users are reliant on the security provided by the cloud provider.
What are the key development considerations when planning Edge AI projects?
Edge AI can steer companies to growth—if they know how to exploit it. Today, few organizations understand how to rapidly design, train, and deploy machine learning (ML) models on edge devices at scale. Organizations that can establish the best edge AI practices and grow their in-house resources are going to be able to shift their strategies far more effectively and get an opportunity to pull ahead and stay there. To get there, they have to overcome the following considerations:
- Time to market. Even the most advanced experts are challenged to verify the size and inference speed of their models against the onslaught of edge devices. Deploying effective ML models on edge devices is difficult. In fact, 90% of all AI models don’t make it into production. Once in production, most models need continual training and updates. Without a trusted and streamlined process, this can be insurmountable. Take the U.S. Navy, which recently overcame the challenge of deploying and updating automatic target recognition models at the speed of operational relevance with the help of the Latent AI Efficient Inference Platform (LEIP). The Navy chose LEIP as the primary development platform due to its capability to accommodate larger models in various environments and to deploy updates over the air rapidly. The result is a 97% decrease in the time it takes to update and redeploy models.
- Scalability. Consistent, trusted tools will allow organizations to accelerate development and scale. Today, nearly every entity is building bespoke capabilities—sometimes multiple bespoke capabilities within a large organization. Flexible tooling will reduce siloed efforts within machine learning operations (MLOps) and across various AI and application teams. Organizations should look for end-to-end platforms with flexible entry points to enable multiple program capabilities, allowing teams to bring their own models and data for optimization or accelerated production from start to finish. LEIP offers this flexibility by providing modular frameworks that allow developers to jumpstart projects at the design phase or bring trained models for optimization and portability to one or more hardware options.
- Design and optimization. Expertly making the size, accuracy, and power trade-offs required to port models to your selected hardware is key to bringing Edge AI to production, which is often the most lengthy and trying part of MLOps. Apprentices and experts alike seek solutions that can improve performance and accuracy better and faster than the experts can. LEIP offers a powerful visualizer that takes the guesswork out of choosing the right model-hardware combination for your project. Leveraging our deep expertise in optimization and performance benchmarking, LEIP provides a library of benchmarked and ready-to-execute configurations that combine model and device optimization with your data in a repeatable process.
- Skillset. Like information technology in cybersecurity, Reuters estimates the skills gap for AI will approach 50% in 2024. In order to maximize operations, a significant part of any organization’s strategy has to be how to expand AI capabilities beyond the data scientists and ML engineers within their organizations. Many look to IT, where nearly 81% of IT professionals feel confident they can integrate AI into their roles right now, but in reality, only 12% have significant experience working with AI. Answering the call, MLOps platform developers are producing low-, or no-code software-as-a-service offerings to ease the AI apprentices into machine learning by eliminating complexity.
- Lifecycle management. Organizations need complete solutions that will help them maintain models in their applications over time. Today, they struggle to retain consistent speed and accuracy with their models across different iterations throughout the ML lifecycle.