Scaling Edge AI in a Data-Driven World, Part One
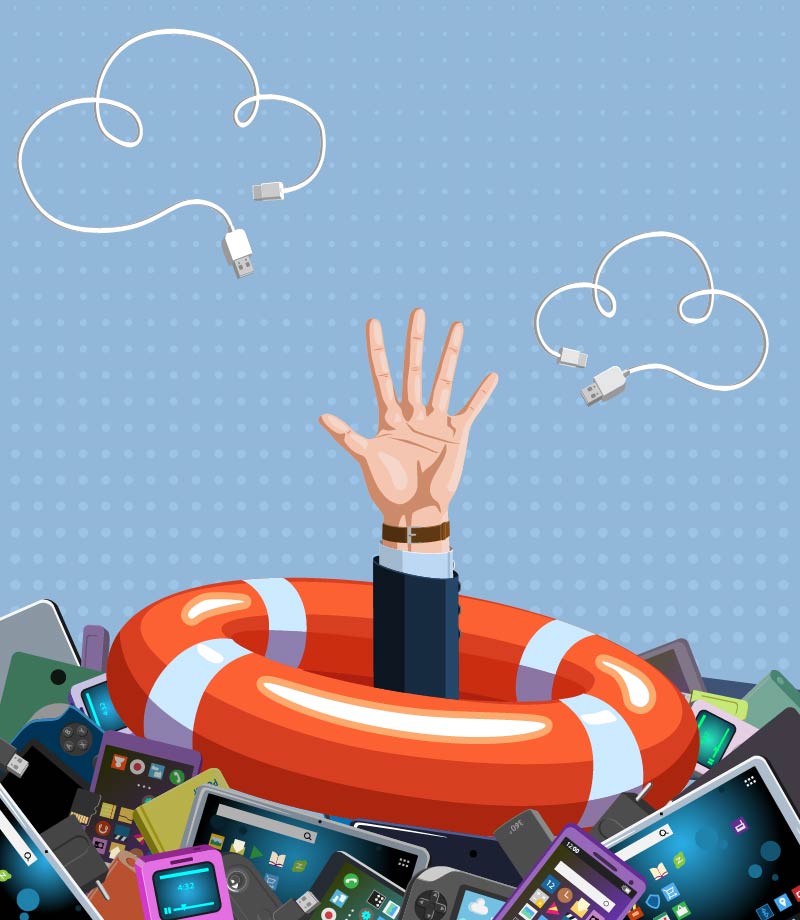
Drowning in Data
We live in a data-driven world where IoT devices are always on, always tracking, and always monitoring. Being perpetually connected also means that we’re producing mind-boggling amounts of data. In fact, IDC Research predicts by 2020 we will be generating 44 trillion gigabytes annually, and the global data sphere will reach 175 zettabytes by 2025. At these almost incomprehensible data levels, we need more than an evolutionary solution to process, store or transport the data. We need revolutionary new ideas.
It’s clear that system solutions relying solely on “cloud-level” processing will not work because those solutions are not practical or efficient to forward and store all edge data for later processing.
Let’s consider the following from a scaling perspective:
- Storage: The world of digital storage can only accommodate 15% of data at that size. Furthermore, edge sensor data is ephemeral and it becomes an IT burden as they are no longer needed.
- Network: Large backhaul infrastructures are built to transport data (e.g. images, video, and other sensor data). However, the network bandwidth is not consistent through the infrastructure and might be variable at the edge, especially in remote or congested areas.
- Processing: Sensor data pose a significant computing workload, and developers look to the cloud with access to large, highly shared data centers. However, it is difficult to scale this centralized solution in a reasonable manner, especially when expecting any near real-time responses.
There are other concerns as well, such as privacy and security. In this data-driven world, businesses understand that “data is king,” and many are reluctant to store their data in the cloud because they perceive it to be outside of their control. Alternatively, smaller on-premise data centers have become an intermediary solution to limit access to their valuable data. However, the challenges around storage, network, and processing persist.
The word on the venture circuit is “edge AI” (and by definition means when AI algorithms are processed locally on a hardware device, without requiring any connection, using data generated and processed directly from the device to give real-time insights in less than a few milliseconds). The reason investors have a heightened interest here is in reaction to the flood of IoT startups entering the market with various edge AI hardware and software solutions. In fact, IoT startups have received $3.6B in funding this year alone.
Cloud vs. Edge, or Cloud + Edge?
Regardless of the market opportunity, it’s not to say that everything should be processed on the edge. Among several advantages, cloud processing offers key benefits in faster deployment for new features. Processing in the cloud also requires less effort to put security patches in play. Many AI developers believe that a hybrid solution with edge computing would be key for scalability.
Cloud vs. edge should never be an either/or proposition. Depending on the application needs, we’ll need both. The cloud enables applications to interact and connect on a large scale. The edge offers spatial proximity between processing and data to afford better efficiency and lower latency. With the increasing number of IoT devices, edge AI is especially useful when real-time information is critical and changes happen quickly. For example, in video surveillance, you want to predict local behavior as it is occurring to catch a culprit, or even prevent an incident.
In our next post, we will do a deeper drive into edge AI scalability issues, and share some longer-term revolutionary ideas shaping the computing landscape. In order to achieve exponential improvements in latency and network bandwidth reductions, we need innovative technologies that break away from the traditional centralized computing schemes to boost application and computing performance. We’ll also highlight how Latent AI can help accelerate your edge AI development and take the hard work out of AI processing on the edge.
Read more: Scaling Edge AI in a Data-Driven World, Part Two
Photo Credits: Adobe Stock