From cloud-first to edge-first: The future of enterprise AI
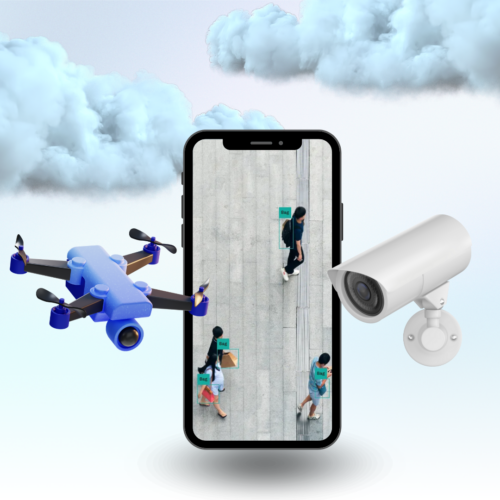
Executive summary
Enterprise AI stands at a pivotal inflection point. While cloud-based generative AI has captured headlines, forward-thinking organizations are recognizing a fundamental shift in how AI must be deployed. This white paper explores why the most innovative companies are transitioning from a cloud-first to an edge-first AI strategy, leveraging lessons from military use cases, industrial applications, and new research on AI implementation challenges and opportunities.
Recent research confirms this trend: 51% of organizations now rank performance (speed and latency) as their most important AI requirement, with 43% specifically valuing edge AI’s ability to process data at its source for real-time analysis. This transition isn’t just about technological preference—it represents a strategic imperative for organizations seeking competitive advantage through AI deployment.
The cloud-centric model has hit its limits
The current cloud-centric model of AI deployment is reaching fundamental limitations:
- Exponential data growth: The average smart factory running AI for defect detection and efficiency analytics generates five petabytes of data per week. Transmitting and processing this volume in the cloud is becoming unsustainable.
- Rising costs: As model sizes and user numbers grow, the costs of developing and running generative AI have escalated.
- GPU constraints: Increased demand has created persistent GPU shortages, driving up infrastructure costs. Organizations competing for limited cloud GPU resources face higher prices and constrained availability.
- Latency requirements: Mission-critical applications require real-time processing that cloud architectures struggle to deliver consistently, especially in environments with connectivity challenges.
Real-world lessons from military and industrial deployments
The US Navy’s Project Accelerated MLOps for Maritime Operations (AMMO) provides a compelling case study for edge-first AI implementation. By modernizing deployed AI at the edge, the project achieved a 97% reduction in model update times—from months to mere days.
This dramatic improvement stems from processing data directly at the source and leveraging enhanced edge compute to update and redeploy rather than transmitting it to centralized cloud infrastructure, where strategic DoD resources would undergo a lengthy process to update, retrain, and provide models back to the edge for deployment.
In combat scenarios where information supremacy determines outcomes, edge AI provides critical advantages. For example, analyzing seabed data for mine detection must happen immediately to create a safe passage for naval fleets. Relying on cloud connectivity and introducing latency in this scenario isn’t just inefficient—it’s potentially catastrophic.
Similar patterns emerge in industrial applications. A major manufacturing company implemented edge AI for quality control using anomaly detection. Initial prototypes running on cloud-connected NVIDIA RTX A5000 proved effective but prohibitively expensive to scale across production facilities. By implementing optimized edge AI, they achieved:
These efficiency gains transformed the economics of deployment, making widespread implementation financially viable while maintaining accuracy requirements.
New research confirms the edge-first advantage
Recent research conducted by TechStrong reveals compelling evidence that organizations are fundamentally reevaluating how they deploy AI, with many finding the edge paradigm perfectly aligned with their core requirements:
- Performance is paramount: 51% of organizations rank performance (speed, latency) as their most important AI requirement, far outpacing other considerations including cost. This marks a significant shift from early AI adoption phases where functionality often trumped performance metrics.
- Real-time processing drives adoption: 43% specifically value edge AI’s ability to process data at its source, enabling instantaneous analysis without network dependencies. This capability becomes particularly critical for applications where delayed insights diminish value exponentially—manufacturing quality control, autonomous systems, security monitoring, and customer experience applications.
- Reliability matters: 39% cite improved reliability as a key driver for edge AI implementation. Systems dependent on continuous cloud connectivity introduce multiple potential failure points—network outages, cloud service disruptions, and bandwidth fluctuations—that edge deployment directly mitigates.
- Latency reduction is critical: 39% highlight reduced latency as a primary advantage.
These findings reveal a nuanced understanding developing among technical leaders: the ideal deployment architecture for AI isn’t universal but context-dependent. The cloud remains optimal for training large models, handling batch processing, and managing vast historical datasets. However, inference workloads—the actual application of AI to generate insights—increasingly belong at the edge where data originates.
The research also uncovered a concerning gap in understanding the energy implications of AI deployment strategies. Only 23% of organizations consider energy consumption an important factor in their AI planning, even though running inference on local devices, like Qualcomm’s Snapdragon X Elite platform, can be 28 times more efficient. This blind spot represents both a missed opportunity for operational savings and an environmental consideration that will likely face increased scrutiny as AI deployments scale.
Additionally, the data showed that edge AI adoption (14%) has nearly caught up to data-center AI deployment (15%), despite being a more recent development paradigm. This rapid convergence signals the beginning of what may become edge dominance for specific AI workloads, particularly as tooling matures to address current implementation challenges.
The customization imperative: One size fits nothing
The research reveals one of the most significant barriers to edge AI adoption: the overwhelming need for customized solutions in environments where computational resources, connectivity profiles, and operational requirements vary dramatically.
- Complete control is non-negotiable: A striking 55% of organizations require complete customization of their edge AI implementations, demanding granular control over model architecture, optimization parameters, and runtime behavior. This level of customization is far beyond what most AI platforms are designed to provide.
- Parameter tuning is essential: Another 40% need the ability to adjust key parameters while maintaining the core model structure. These organizations need frameworks that expose critical configuration options without requiring complete rebuilds.
- Off-the-shelf solutions fall flat: Only 5% find standard, pre-packaged solutions sufficient for their edge AI needs. This reveals a fundamental mismatch between current product offerings and market requirements.
This extraordinarily high demand for customization (95% requiring some level of adaptation) reflects the diverse and specific requirements of edge deployments. In cloud environments, standardization is easier—resources are elastic, networking is consistent, and hardware is homogeneous. Edge deployments face the opposite reality: fixed resources, variable connectivity, and heterogeneous hardware across installations.
Unfortunately, current tooling falls dramatically short of meeting these needs, with 52% of organizations expressing dissatisfaction with available edge AI development tools and platforms. Only 17% report being “very satisfied” with current solutions. This satisfaction gap represents one of the most significant market opportunities in the AI ecosystem.
The customization imperative extends beyond mere technical preferences—it reflects fundamental business requirements:
- Hardware heterogeneity: Organizations deploy edge AI across diverse hardware, from powerful edge servers to resource-constrained embedded devices, each requiring different optimization techniques.
- Variable performance targets: Different applications have distinct latency, throughput, and accuracy requirements that must be precisely balanced.
- Dynamic operating conditions: Edge environments experience fluctuations in available power, connectivity, and computational resources that models must adapt to in real-time.
- Domain-specific constraints: Industry-specific requirements around safety, determinism, and explainability often necessitate specialized model architectures and runtime behaviors.
The implications are clear: vendors offering one-size-fits-all edge AI solutions are fundamentally misaligned with market requirements. Successful platforms must provide comprehensive customization capabilities while simultaneously reducing the expertise required to implement those customizations—a challenging but essential balance to strike.
Powering the edge AI revolution: Economic impact
The economic advantages of edge AI extend far beyond theoretical benefits, potentially transforming the ROI calculations that have historically slowed AI adoption. A detailed analysis of real-world manufacturing implementations reveals the scale of these advantages and their implications for enterprise AI strategy.
Case study: Anomaly detection in manufacturing
A large manufacturing enterprise sought to implement computer vision-based anomaly detection to improve yield and reduce production waste across multiple facilities. Their initial implementation used standard models running on high-performance NVIDIA RTX A5000, with plans to run 100 video streams concurrently in the production pipeline.
After optimizing these models for edge deployment using advanced quantization techniques, they achieved remarkable efficiency improvements:
- Memory footprint collapsed: Memory utilization reduced from 14.1GB to 3.8GB per model instance—a 73% reduction that fundamentally changed scaling economics.
- Processing speed surged: Inference time decreased from 55.2ms to 14.7ms—a 73% improvement, enabling real-time processing of high-frame-rate video streams.
- Hardware requirements plummeted: GPU requirements reduced from 50 cards to just 4—a 92% reduction in hardware needs.
- Capital expenditure transformed: At approximately $4,500 per GPU, this represents a reduction from $225,000 to $18,000 in hardware costs—shifting the project from capital-intensive to economically scalable.
Most importantly, these dramatic efficiency gains came with negligible impact on model effectiveness. The edge-optimized models maintained an Area Under ROC (AU-ROC) score of 0.99127 compared to the original model’s 1.0—a difference that had no material impact on operational outcomes.
Beyond hardware: The total economic picture
The direct hardware savings represent only the beginning of edge AI’s economic advantages:
- Energy consumption: Edge-optimized AI typically reduces energy requirements by 65-80% compared to cloud-based alternatives, translating to substantial operational cost savings and improved sustainability metrics.
- Network costs eliminated: Processing data locally eliminates data transfer costs that can reach hundreds of thousands of dollars annually for bandwidth-intensive applications like video analytics.
- Operational continuity: Reduced dependency on network connectivity translates to fewer operational disruptions, with some organizations reporting a 40-60% reduction in AI system downtime after transitioning to edge-optimized architectures.
The scalability equation
Perhaps most significantly, edge-optimized AI fundamentally changes the scalability equation. Organizations that previously found AI deployment economically viable for only their most critical applications can now justify broader implementation:
- Deployment breadth: Manufacturing companies previously limiting computer vision deployment to critical quality control points can now economically monitor entire production lines.
- Geographic distribution: Retailers originally implementing computer vision only in flagship locations can now cost-effectively deploy across their entire store network.
- Application depth: Organizations that justified AI only for primary use cases can now implement secondary and tertiary applications on the same infrastructure, extracting more value from existing investments.
This economic transformation shifts AI from a specialized tool for high-value applications to a ubiquitous capability embedded throughout operations—the true promise of AI that has until now remained elusive for most organizations.
How organizations should prepare
Organizations seeking to capitalize on the edge-first AI revolution should take several key steps:
1. Invest in edge-ready infrastructure
Build or upgrade IT infrastructure to support decentralized computing, including edge servers, IoT devices, and robust connectivity solutions. Prioritize scalability, low-latency, and data processing capabilities at the edge.
2. Develop edge-optimized applications
Re-architect applications to leverage edge computing. Focus on lightweight, modular designs with AI/ML capabilities tailored for real-time processing and local decision-making at the edge.
3. Enhance data security and governance
Strengthen data protection frameworks, including encryption, authentication, and access controls, to address the increased data dispersion at the edge. Ensure compliance with regulations for edge-based data processing.
4. Implement proper development tools
Select development platforms that address the current tooling gap. Look for solutions that offer:
- Model optimization capabilities
- Hardware-aware deployment tools
- Flexible customization options
- Automated quantization and pruning
- Security features including model encryption and watermarking
5. Balance cloud and edge resources
While shifting to an edge-first mindset, maintain cloud resources for training, orchestration, and coordination. Design architectures that leverage the strengths of both paradigms.
Conclusion: The future is edge-first
The parallels to cloud computing’s rise are striking. Just as organizations initially resisted moving workloads to the cloud before embracing it as inevitable, edge-first AI will follow a similar trajectory. But this shift will happen faster because the driving forces—data volume, latency requirements, security concerns, and costs—are already at critical levels.
Organizations became data companies over the past two decades with insights being drawn utilizing data warehouses over several months. Real-time insights will help organizations respond to their customers efficiently and effectively. The shift to the edge is the only economical way to win in our increasingly data-intensive world.
The future of AI isn’t just about bigger models or more computing power in the cloud. It’s about bringing intelligence closer to where data is generated and decisions need to be made. 2025 is the year this becomes impossible to ignore.