Gartner Recognizes Latent AI as Unique Edge AI Tech Innovator
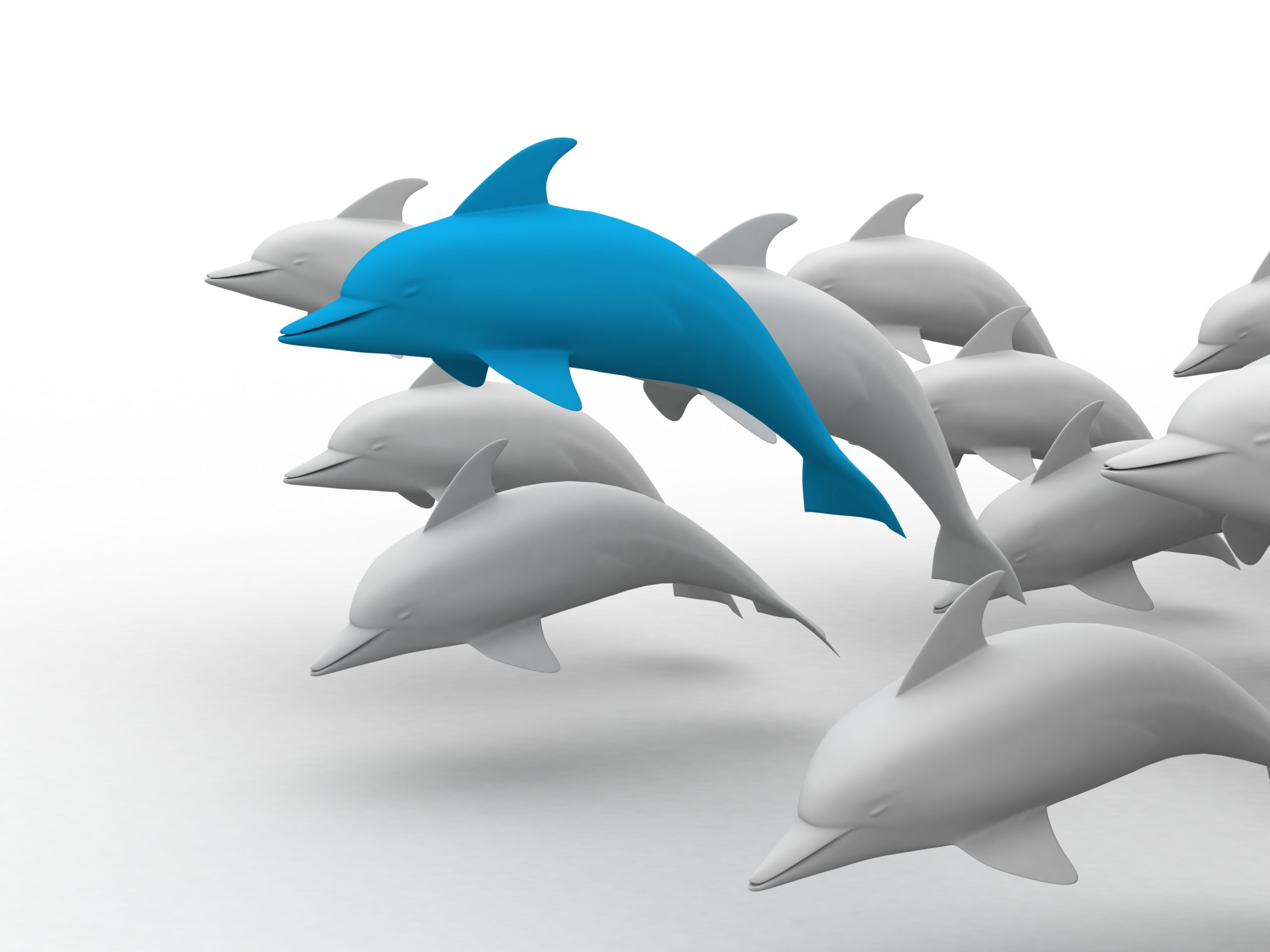
Gartner recently published a new report on Tech Innovators in Edge AI, covering the trends and impact of Edge AI on products and services by analysts Eric Goodness, Danielle Casey, and Anthony Bradley. Latent AI greatly appreciates Gartner’s interest in Latent AI and coverage in this analysis.
The following is a summary of the report related to Latent AI technologies to help embedded developers and AI engineering teams identify adaptive edge AI software solutions that bring their AI models to the edge, faster, and less expensively.
The Edge AI Market Dynamic
Business outcomes enhanced and influenced by artificial intelligence (AI), machine learning (ML), and deep neural networks (DNN) are changing the landscape of value creation in IT and OT systems. While distributed compute (from the cloud down to the network/Telco/access edge to the gateway/appliance/device and sensor edges) is here to stay, “on-device” AI at the edge continuum is significantly disrupting this value creation equation. Traditional cloud-based compute workloads are increasingly migrating to the edge, where most of the data is generated, driven chiefly by latency and bandwidth cost optimizations.
Latent AI and other edge AI software enablers and innovators prove to be the catalysts for advancing adaptive AI technologies, and until now, running AI on the severely constrained edge has been a demanding process at best. Cloud trained AI and ML models cannot be inferred at the edge by force-fitting due to stringent performance, power consumption, size/footprint, low-latency, high-bandwidth connectivity, privacy, and security constraints.
Latent AI has addressed this challenge head-on by enabling sophisticated model optimizations for compute, energy, and memory without requiring changes to existing AI/ML infrastructure and frameworks. AI model optimization for inference at the edge is critical to IoT enabled products and services in a wide swath of “connected” verticals, including automotive, healthcare, financial, energy, manufacturing, media, and other real-time applications. Moving forward, the adoption of IoT will increasingly depend on edge AI capabilities.
Among twelve other “2020 Edge AI Tech Innovators,” Latent AI is proud to be included for our unique solution.
Edge AI Strategic Implications to Consider Now
The impact of edge AI on new products, services, and solutions over the next decade will require the use of end-to-end tools for managing the ML lifecycle and workflow for model inferencing (MLOps) across the edge continuum.
By 2025, more than half of all data analytics with DNNs will happen at the edge, where the data is generated. Further, edge AI services will overtake the cloud for large managed service providers and Telcos.
There are over 250 billion microcontrollers in the world today. By 2025, more than 50% of microcontroller-based embedded products will ship with on-device AI capabilities, such as those powered by tinyML. These dynamics open the market and expand embedded and IoT systems’ opportunities to perform tasks that have been impossible to accomplish until now.
Container-based architectures will be the foundation for most AI pipelines since edge devices are heavily resource-constrained. Containers are lightweight and monolithic applications can be broken down into smaller and meaningful tasks to run as microservices on edge devices. This allows for efficient usage of compute and network resources. The portability of containers also lends well to the continuous integration/continuous delivery (CI/CD) paradigm, where embedded systems need to be updated frequently when AI models are refined.
Why Latent AI is an AI Innovator
Gartner segregated the edge AI vendors into practical, unique, and disruptive categories based on innovation use-case research. Latent AI, categorized as unique given our ability to provide high-performance compression for dynamic AI runtime workload management and edge model training, earned us a ranking in the top twelve featured vendors.
The Latent AI Efficient Inference Platform (LEIP SDK) enables developers to automate compression and compilation of DNNs for inference in edge-constrained devices powered by a wide variety of processors from the sensor to the network/Telco/access edge.
LEIP consists of three modules: LEIP Compress, LEIP Compile, and LEIP Adapt.
-
- LEIP Compress is a quantization optimizer for edge devices and automates exploration of lower bit-precision AI training (INT8 and below) to deploy efficient DNNs. This allows for a trade-off between accuracy, model size, power consumption, and performance of AI models.
- LEIP Compile integrates AI training and a compiler into an automated framework to generate optimized code based on the DNN model, parameters, and available hardware resources.
- LEIP Adapt allows dynamic AI workload processing at runtime for improving inference efficiency while maintaining model performance accuracy.
Most complex AI models are trained in the cloud on high bit-precision GPUs such as single-precision IEEE FP32, allowing for almost unrestricted compute resources to produce desired outcomes. But, while inferencing an AI model on the resource-constrained edge, 8-bits (INT8) or less is sufficient (this offers a 4X compression). However, LEIP produces 10X compression results with only a 1% loss of accuracy.
Real World Use Cases
Auto OEM
A tier one automotive OEM has partnered with Latent AI to develop in-cabin and rear-facing cameras specifically to enhance physical safety and to meet regulatory compliance. For example, gaze monitoring applications driven by edge AI models can alert drivers and fleet operators to distractions such as eating, texting, or drowsiness while driving. These new features will improve safe driving behavior, help to ensure employee safety, and compliance enforcement.
Content Delivery Networks
When AI services are distributed away from the cloud and closer to the service edge, the overall application speed increases without moving data back-n-forth. Deploying edge AI services is an arduous task, as AI is both computational and memory-intensive. AI models need to be tuned so that the computational latency and bandwidth are radically reduced for the edge.
For example, Cloudflare’s edge networks already serve a significant proportion of today’s internet traffic and serve as the bridge between devices and the centralized cloud. High-performance AI services are possible because distributed processing has excellent spatial proximity to the edge data. Using WASM to perform architectural explorations with LEIP focuses the achievable performance on the available edge infrastructure. With exceedingly compressed/optimized WASM neural networks, distributed edge networks can move the inference closer to users, offering new edge AI services proximal to the data source.
Smaller models enable distributed AI services, resulting in applications that can run without access to deep cloud services, such as a doorbell or doorway entry camera connected to Cloudflare Workers service to verify if a person is present in the camera’s field of view.
Impact of Latent AI
Gartner states that powerful tools and platforms such as LEIP expedite the deployment of advanced edge AI solutions while driving wider adoption and market growth. The flexibility and powerful optimizations of the LEIP SDK allows fast experimentation with model accuracy and a set of critical edge constraints, leading to better tradeoffs (cost vs. complexity) and accelerating time to market for application developers. LEIP takes the hard work out of manually pruning and tuning models for inference at the edge, enabling speed to market.
Summary
With the proliferation of inexpensive sensors, pervasive connectivity, and the ubiquitous internet, petabytes of data generated all around us have exponentially increased the complexity of big data at the edge. Moving all of this data to the cloud for processing, running AI models, and analytics no longer makes sense. The sheer cost of data communication, services for processing, analytics, and storage in the cloud can render a solution cost-prohibitive.
Latent AI accelerates this edge AI revolution by offering a tools platform to automate AI model compression, compilation, and deployment at the edge continuum. LEIP SDK is available in a Docker container and can be used in any developer environment. The data doesn’t leave the premises and the SDK integrates with a standard workflow.
Latent AI helps unlock tremendous business value for product and solution developers in the edge AI space, and we’ve only scratched the surface.
Vasu Madabushi, Product Marketing Consultant and Guest Blogger
Images: Gartner, Latent AI, Adobe