Why smaller AI is still important in the age of bigger computers
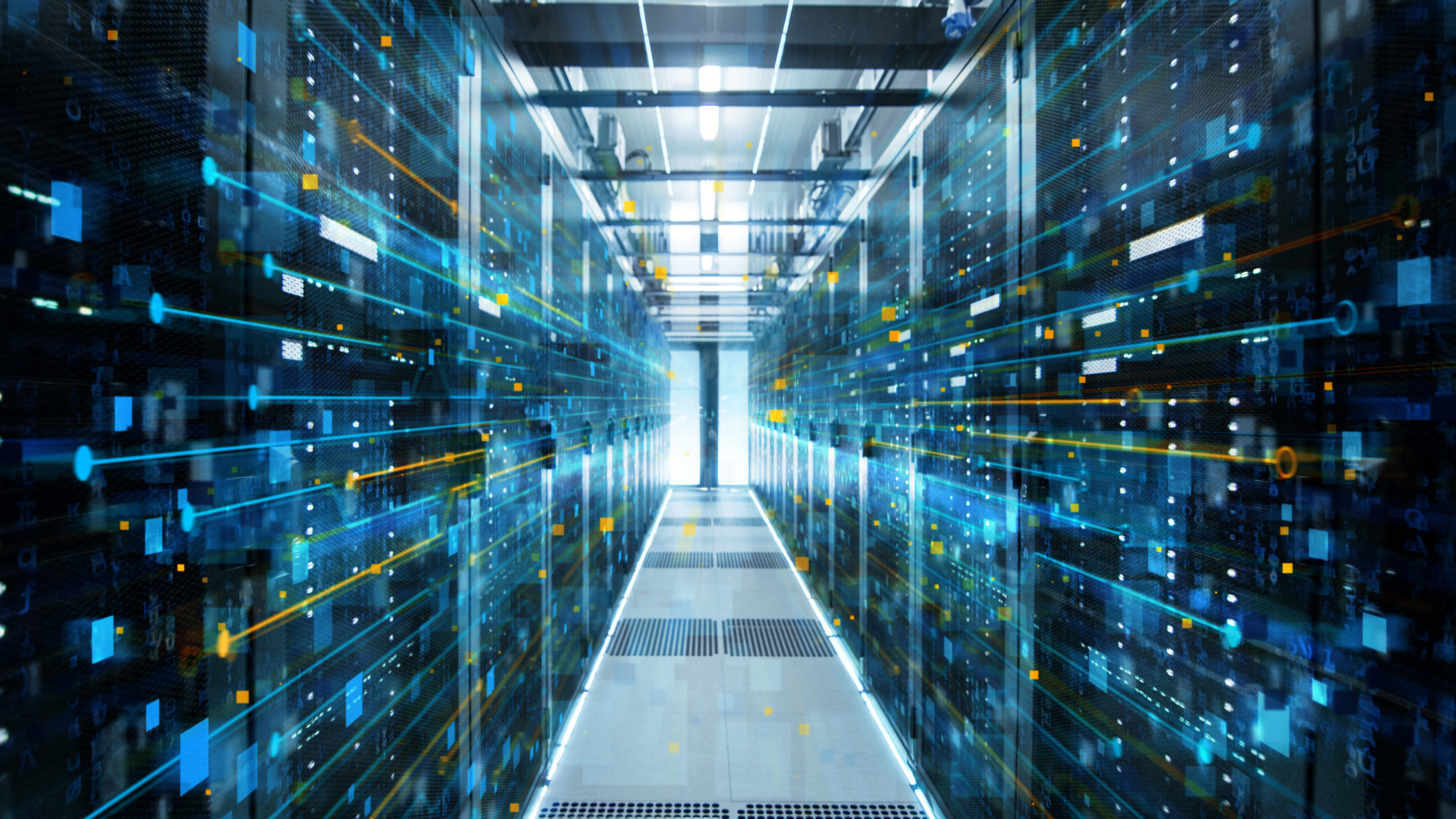
What happens to our business if nobody needs bigger, faster computer processors?
This was the quiet question keeping computer chip executives awake at night in the early 21st Century. It wasn’t that they were hitting a technical ceiling: their engineers continued to defy “Moore’s Law is dead” doomsayers, cranking out faster and faster chips year after year. No, it was that the companies were running out of demand for those faster chips. Saying that Microsoft Word can run 50% faster than last year is great only if the customer feels that Word is too slow. Eventually, the computer architects would build a chip good enough to run MS-Word at a speed that would satisfy everyone. And then nobody would want to buy the new chip. What happens then?
Then, AI happened.
Artificial Intelligence (AI) models exploded onto the scene with breakthroughs like Alex Krishevsky’s AlexNet. In 2012, AlexNet was entered into the international ImageNet Large Scale Visual Recognition Challenge, a competition to see which of the newest, shiniest computer vision techniques could correctly identify the most animals and everyday objects in a large database of images. AlexNet dominated the competition, winning by an unthinkable 10.8% accuracy margin over the runner-up. It was obvious that AI models were the way of the future, and they would need all the compute they could get. And the stressed-out industry executives could finally get some sleep because their products were needed again, badly.
AI models demand computing grunt. A lot of it. State-of-the-art models need to load billions of parameters and run trillions of computations each second. That means hours, days, or sometimes weeks spent training. Even for deployment, trained AI models often require specialized hardware like Graphics Processing Units (GPUs) or accelerators just to handle the intense workload, especially for embedded applications at the edge. In today’s AI race, any additional processing speed squeezed out of a chip by the wizards in the semiconductor business will be immediately soaked up by even more resource-hungry AI models.
But this produces another problem:
What happens if you need – but can’t have – bigger, faster computer processors?
The newest processors running the smartest AI models cost thousands of dollars and suck back hundreds of Watts of power when they run. That effectively prices the latest AI out of reach for many consumers, causes environmental and energy waste concerns for our electric grid, and generates endless headaches for the many companies trying to fit AI models affordably into their products.
That’s why smaller AI remains important even in the age of bigger computers. We have to figure out how to make AI smaller and faster without requiring you to shell out for the latest processors. That’s why Latent AI was created. The Latent AI Efficient Inference Platform (LEIP) allows you to either run one of our speed-tuned models or compress and quantize your own model by up to 10x, letting it race up to 2-3x faster, while using up to 90x less energy. These benefits can happen on any device you want, from a smart home appliance to an Android phone, from an autonomous vehicle on the highway to a jet breaking the sound barrier.
And to the semiconductor executives: we promise we’re not after your 8 hours a night.
Read more about how LEIP gives organizations what they’ve been missing – a single tool that provides a repeatable and scalable path for delivering optimized edge models quickly.
To learn more, visit latentai.com or contact us at info@latentai.com