Continual object detection in real-time: Adapting to an ever-changing world on the edge
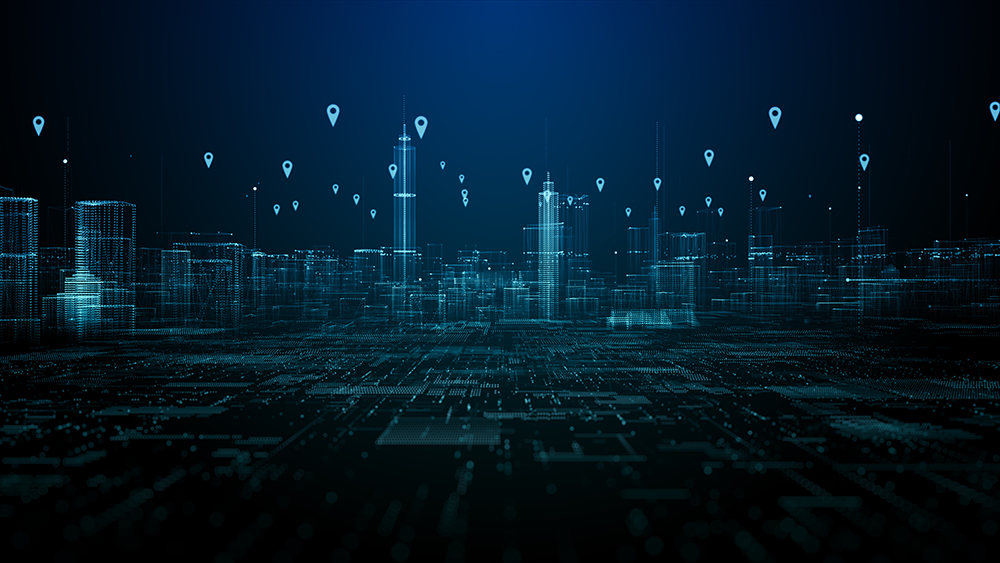
Instant decision-making and processing is critical in missions where the slightest delay can have significant consequences. Consider search and rescue (SAR) teams entering disaster zones with limited and incomplete information. As the crisis unfolds, situations can change rapidly. This would require the team to quickly adapt their classifications by also accommodating entirely new elements. Without up to date information, SAR teams can’t make informed decisions, which hampers their ability to respond.
The dynamic process of real-time continual object detection not only necessitates continuous identification and precise localization of objects, but also demands the ability to account for changing conditions and the presence of previously unknown objects. In the realm of artificial intelligence (AI), incremental classification tasks have long been the focus of research and development. However, continual object detection combines both localization and classification simultaneously, and is of greater importance to real-world applications that extend well beyond SAR missions to autonomous vehicles, surveillance systems, etc. This task presents unique challenges, as it requires the model to not only recognize known objects but also identify and adapt to previously unseen or unlabeled objects. Unfortunately, this is less explored although it has great potential. Due to its application across various domains, real-time continual object detection can revolutionize the way we interact with the world around us.
Enhancing Awareness for Immediate Response:
Continual object detection is of utmost importance in applications that require quick responses, such as autonomous vehicles and surveillance systems. AI systems can make split-second decisions, while continuously analyzing and understanding their surroundings, and ensuring safety and efficiency. Whether it’s detecting potential road hazards or suspicious activity, the ability to localize and classify objects in real-time becomes critical.
Security and Anomaly Detection:
Real-time continual object detection serves as a valuable asset in the domains of safety and security. Through seamless integration with anomaly detection systems, it becomes possible to track intruders or identify hazardous anomalies in a prompt manner. Furthermore, real-time detection enables the identification of new types of objects that may pose potential risks. This capability empowers us to avoid dangers and take precautionary measures, contributing to overall safety and security.
Broader Understanding of the Real World:
Systems equipped with real-time object detection capabilities possess a deeper understanding of the dynamic real world. By continuously processing data from streaming sources, these systems adapt and learn on the fly. This adaptability is particularly advantageous for unmanned systems (UxS) such as airborne and underwater drones (UAVs and UUVs), robots, and other applications that interact with objects. With an improved understanding of objects and their contexts, these systems can operate more efficiently and effectively.
An agent exploring the surroundings, identifying previously unseen object classes in real-time. Dataset Source: https://oakdata.github.io/
Empowering Edge Computing:
The integration of real-time object detection with edge computing opens up a multitude of possibilities. Sensors and systems at the edge encounter many dynamic situations that are not available during training time. Here are some examples of new objects and their variants: (a) a construction crew mending a pothole, (b) police and emergency vehicles in the scene, (c) changes in the environment due to adversarial attacks. By enabling continual learning on the edge, processing can be done locally, without the need to transfer sensitive data off the device. This localized data processing not only enhances privacy but also reduces latency and bandwidth requirements, ensuring mission success even in air-gapped environments.
The efficient edge deployment capabilities offered by Latent AI provide a solid foundation for the integration of real-time continual object detection. This combination paves the way for real-time, localized, and adaptive object detection systems. Latent AI’s Efficient Inference Platform (LEIP) enables the optimization of runtimes for edge computing applications. LEIP-optimized neural network runtimes designed specifically for targeted edge hardware would ensure efficient execution of these continually learning systems, enabling incremental learning and adaptation techniques in real-time. These portable models optimized for compute, energy and memory usage provide the perfect foundation to explore how this feature can be manifested on the edge.
LEIP recipes serve as a solid foundation to rapid prototyping, and recipes specifically tailored for object detection tasks can be further customized to accommodate changing conditions and the presence of previously unseen objects. By utilizing customizable recipes, incremental learning techniques, hardware optimization, and edge computing capabilities, Edge AI, optimized with LEIP, is invaluable for identifying suddenly appeared objects or augmented instances of existing objects.